Resources
From expert advice and commentary to guides, reports, videos and more, dive into a wealth of industry wisdom curated by retail and merchandising specialists.
Browse resources
Guide 
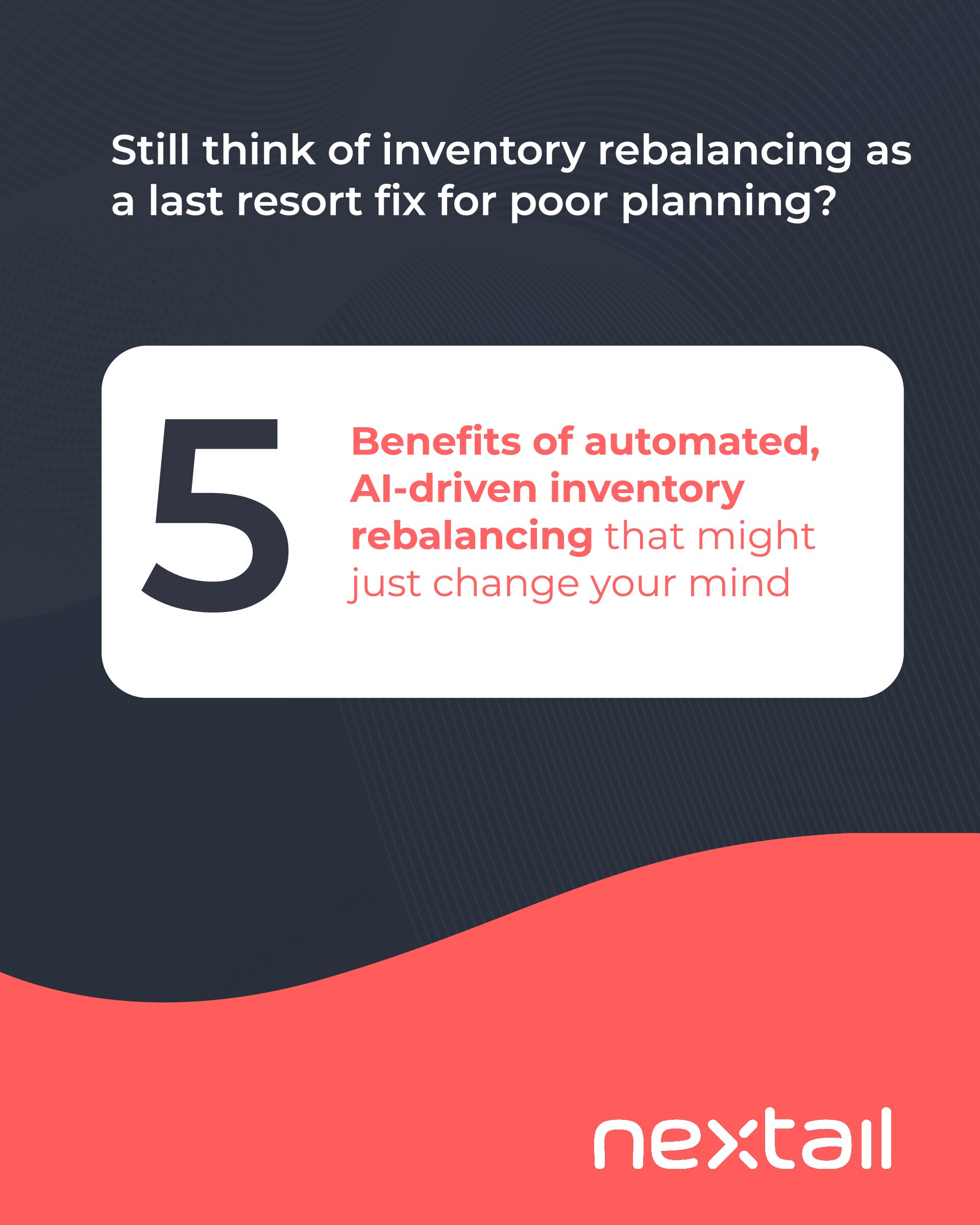
5 benefits of AI-driven inventory rebalancing
Read more
Blog 
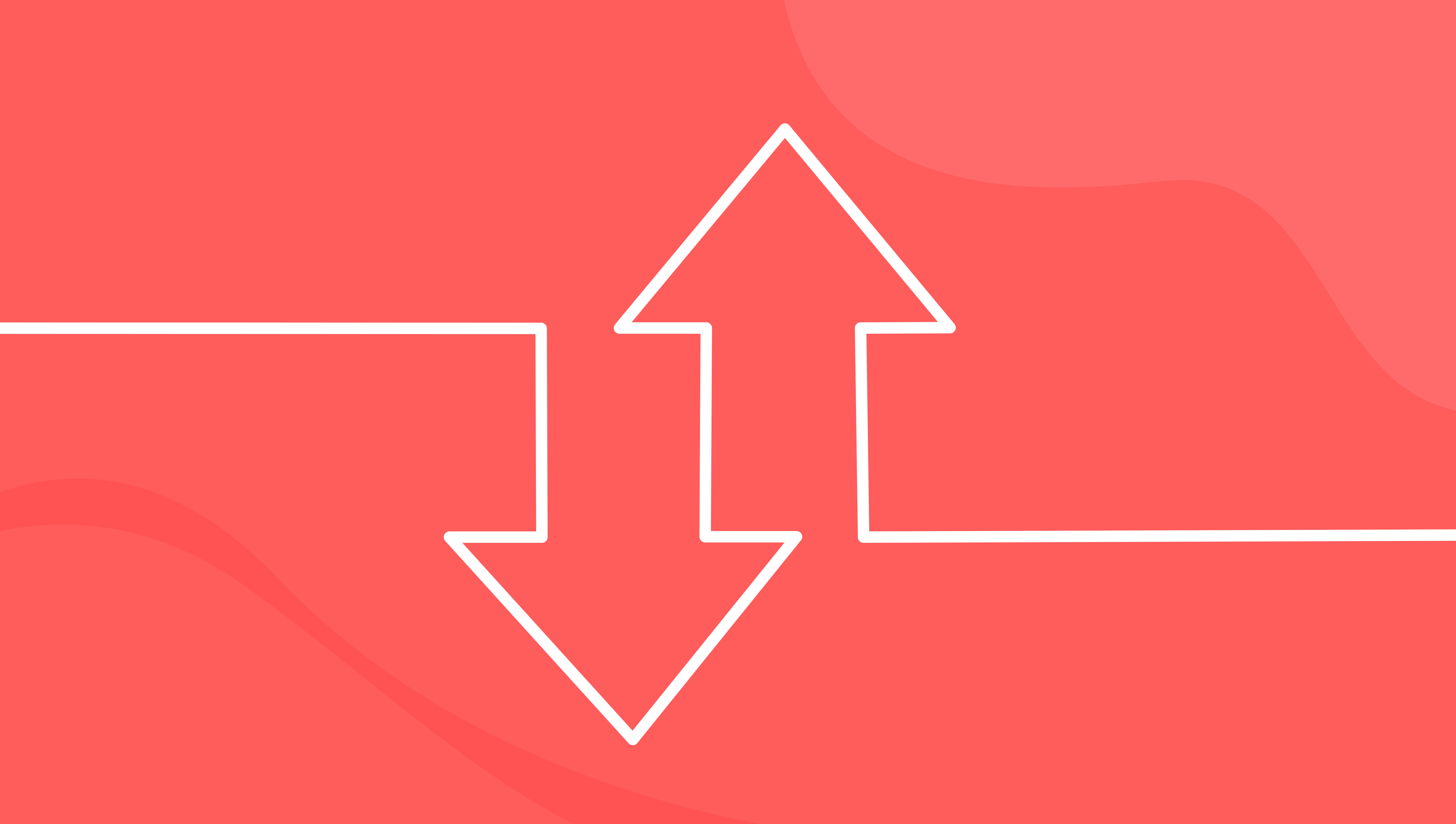
Understanding the basics: A guide to “bottom-up” hyper-local merchan...
Read more
Blog 
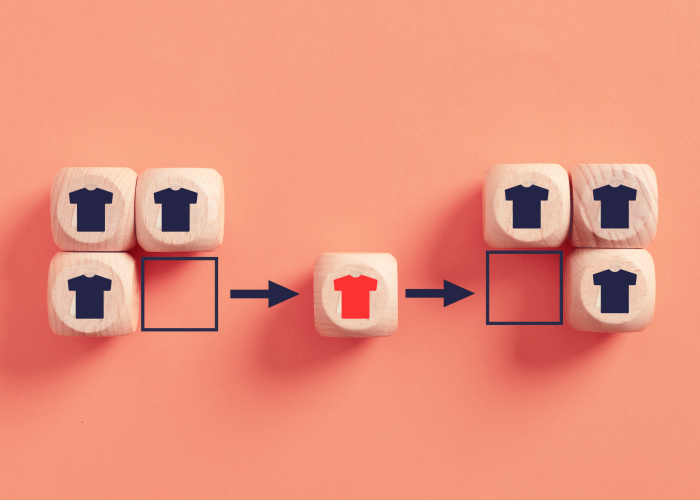
From pain point to profit: The power of inventory rebalancing in fashion
Read more
Blog 
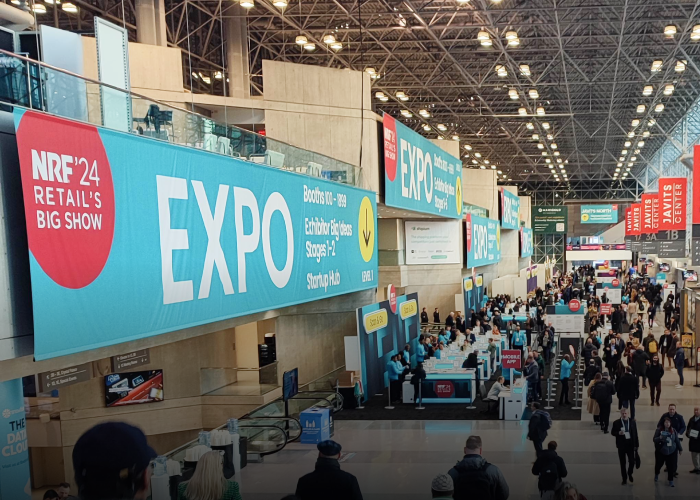
Retail AI in 2024: 3 key takeaways from NRF’s Big Show
Read more
Blog 
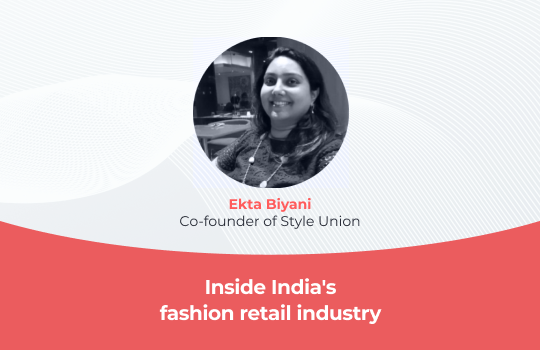
Inside India’s fashion retail industry with Ekta Biyani, Co-founder of Style U...
Read more
Blog 
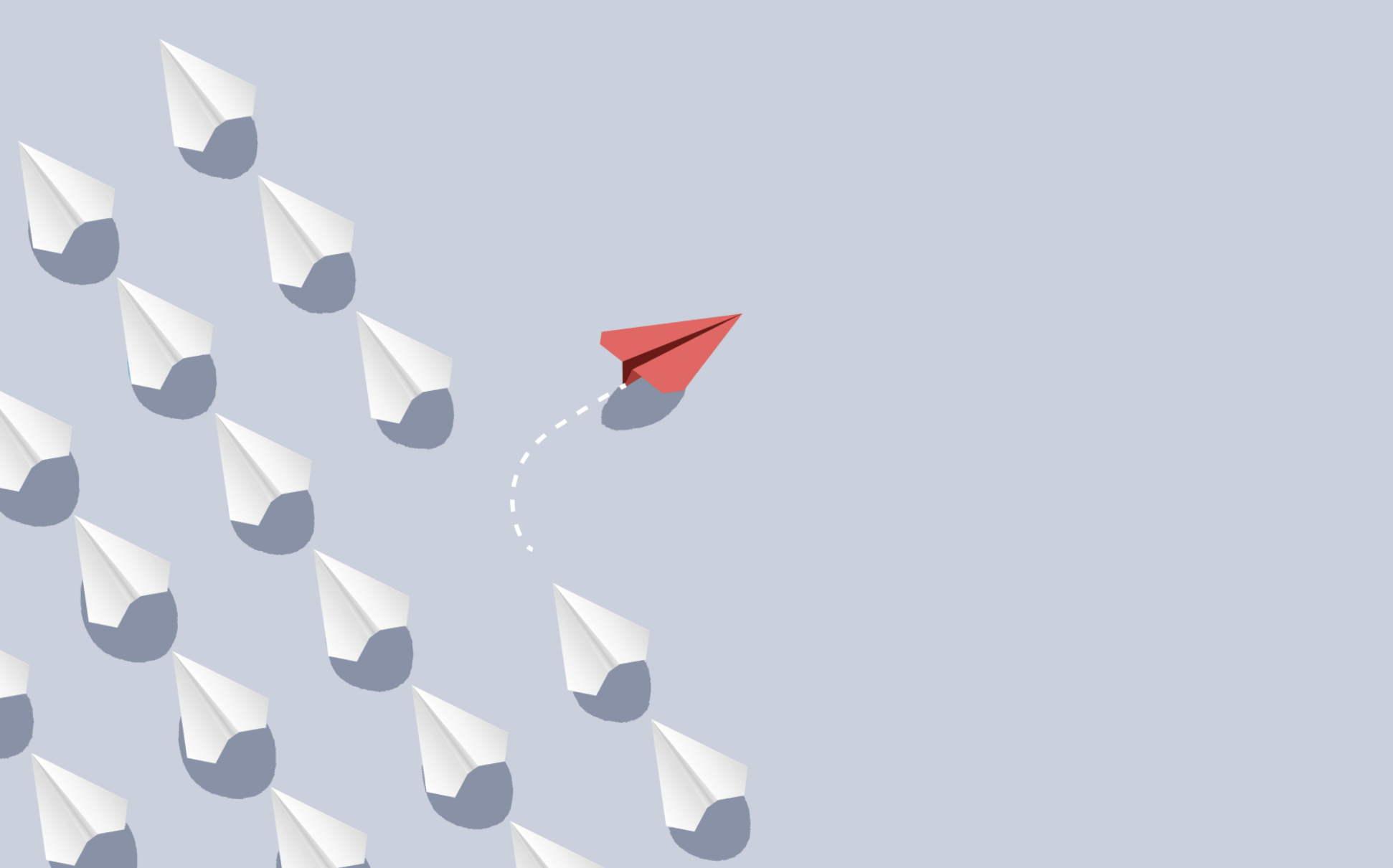
Maximizing margins: Why fashion retailers need to rethink inventory in 2024
Read more
Guide 
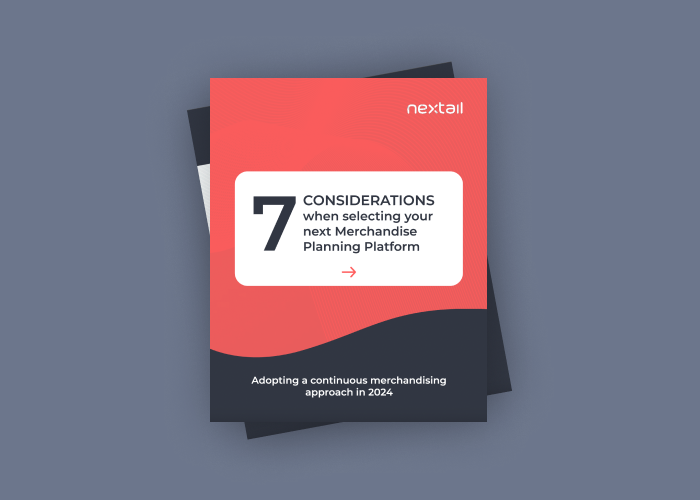
7 considerations for selecting your next merchandise planning platform
Read more
Blog 
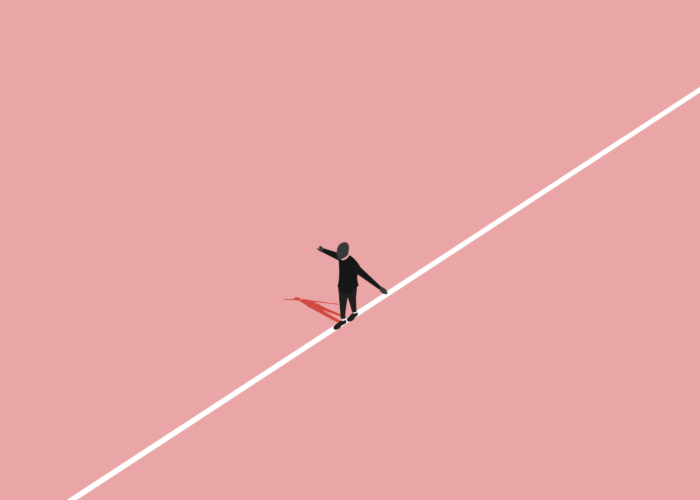
Finding the right balance: Maximizing retail headcount and technology in fashion
Read more
Blog 
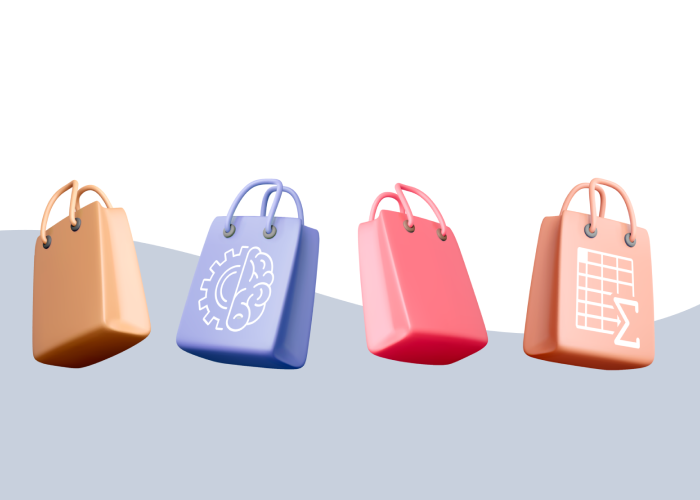
The evolution of assortment planning in fashion retail: From spreadsheets to AI
Read more
Guide 
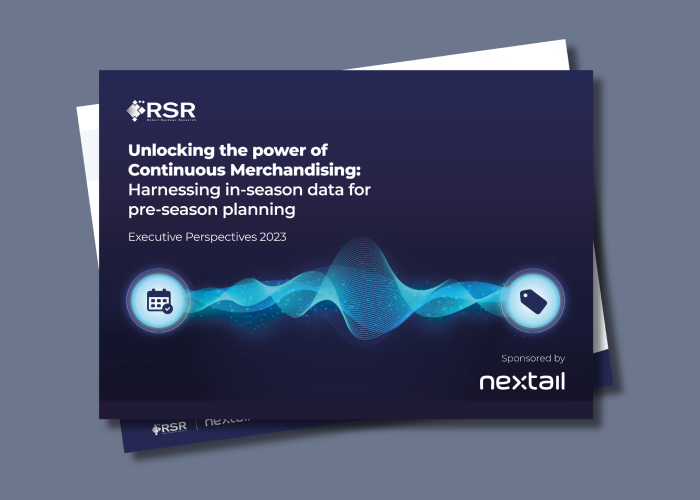
RSR Guide to continuous merchandising
Read more
Blog 
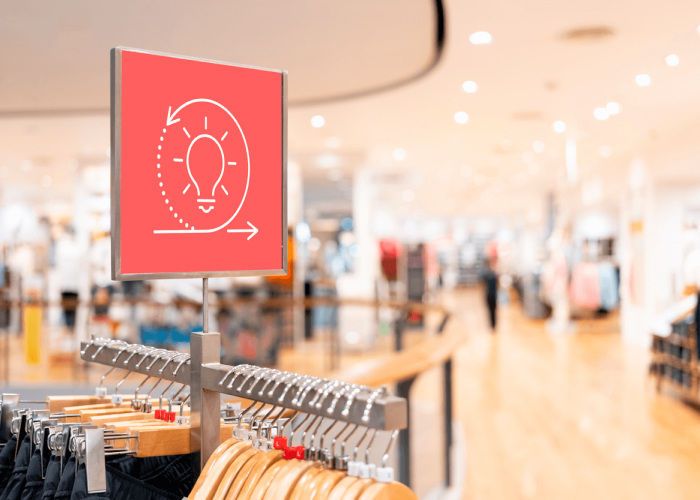
Why fashion retailers need to rethink the traditional planning cycle
Read more
Guide 
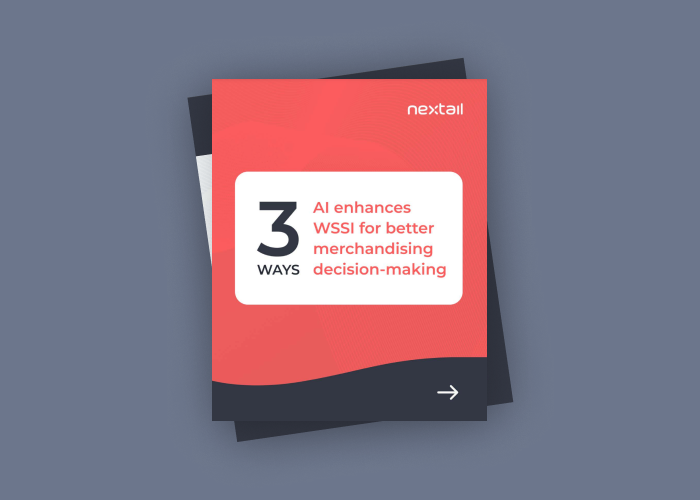
Weekly sales, stock and intake (WSSI) guide
Read more
Blog 
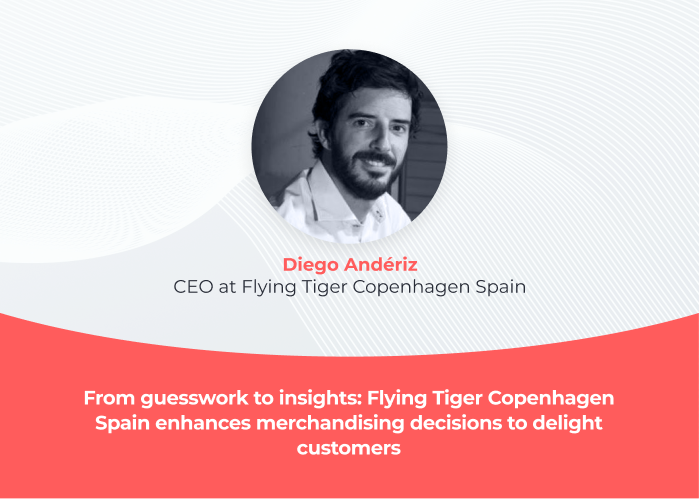
From guesswork to insights: Flying Tiger Copenhagen Spain enhances merchandising...
Read more
Blog 
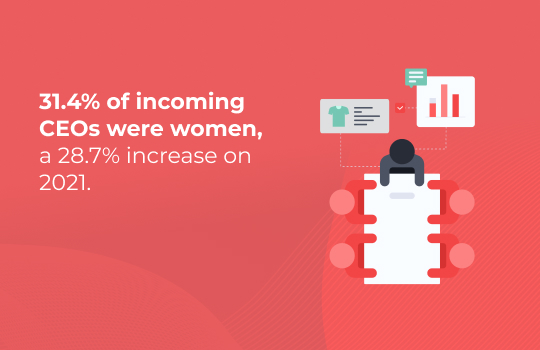
Fashion brands are choosing more female CEOs to represent them in the boardroom
Read more
Blog 
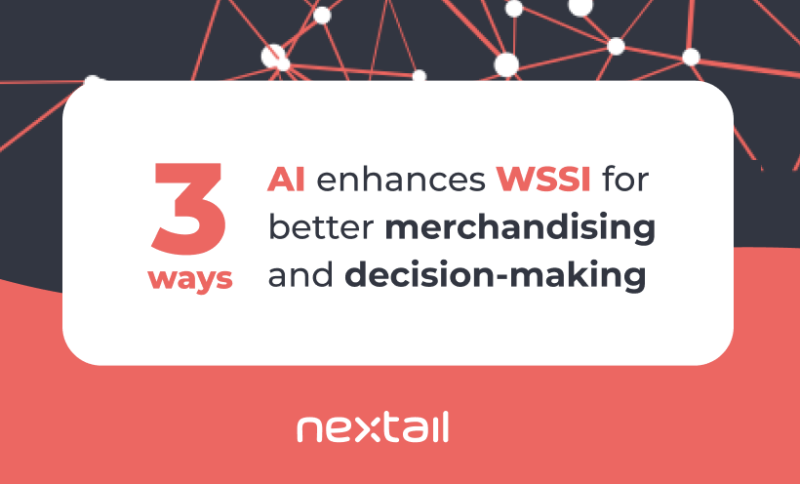
Advanced retail tech picks up where WSSI leaves off
Read more
Ready to see
real results?
Let us show you how Nextail can help drive profitable growth across your entire network of stores and channels.
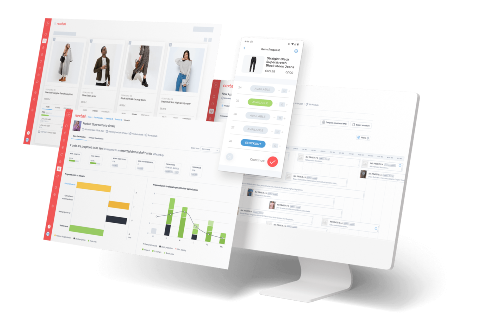